The End of Brute Force AI: A New Era of Reasoning and Human-like Intelligence (Meta Description: Future of AI, Reasoning AI, Ilya Sutskever, NeurIPS, Post-Pre-training AI, AI prediction)
Whoa, hold onto your hats, folks! The AI world just got a whole lot more interesting. Ilya Sutskever, the co-founder and former chief scientist of OpenAI, dropped a bombshell at NeurIPS 2023 in Vancouver. He declared the era of "pre-training" – that is, brute-forcing AI with mountains of data and colossal computing power – is officially over. This isn't just some techie whispering in a dark corner; this is a seismic shift, a paradigm change that's going to reshape the very fabric of artificial intelligence as we know it. Think about it: for years, we've been throwing massive datasets and computational resources at AI models, hoping they'd magically learn. We've been building bigger and bigger brains, hoping for smarter and smarter results. But Sutskever's proclamation suggests we've reached the limits of that approach. We've hit a wall. Now, what? The answer, according to him, lies in a future where AI actually thinks – where it reasons, infers, and understands, much like a human brain. This isn’t just about incremental improvements; it's about a fundamental leap forward, a transition from brute force to genuine intelligence. Forget those mind-numbingly large language models (LLMs) we’ve been obsessed with; the future is about creating AI systems capable of nuanced thinking, creative problem-solving, and adaptation to unpredictable situations. This isn't just a prediction; it's a challenge, a call to action for the entire field of AI research. This article delves deep into Sutskever's statement, exploring its implications, the challenges ahead, and the thrilling possibilities that lie in store. Get ready for a mind-bending journey into the future of AI, because things are about to get really, really interesting. Are you ready to explore the uncharted territory of reasoning AI? Let’s dive in!
The Dawn of Reasoning AI: Moving Beyond Pre-training
Sutskever's statement at NeurIPS wasn't just a casual observation; it was a culmination of years of research and a growing understanding of the limitations of the current pre-training paradigm. For years, the AI field has been obsessed with scaling up: bigger models, more data, more compute. This "bigger is better" approach, while yielding impressive results in certain areas, has fundamental limitations. It's like trying to build a skyscraper without a solid foundation – eventually, it’ll collapse under its own weight.
The pre-training approach, while effective in tasks like language translation and image recognition, falls short when it comes to complex reasoning tasks. These models are essentially pattern-matching machines, identifying statistical correlations in massive datasets. They can generate impressive text, translate languages, even create art, but they lack genuine understanding. Think of it like a parrot that can perfectly mimic human speech without comprehending the meaning. Impressive, yes, but hardly intelligent.
The shift towards reasoning AI implies a fundamental change in how we approach AI development. Instead of simply feeding models vast amounts of data, we need to focus on developing algorithms that can reason, infer, and learn from fewer examples. This requires a deeper understanding of cognitive processes and the development of novel architectures that mimic the way the human brain processes information.
This isn't just about building smarter algorithms; it's also a question of ethical considerations. As AI systems become more powerful and capable, it's crucial to ensure they are aligned with human values and goals. A reasoning AI, with its capacity for greater autonomy and decision-making, requires even more careful design and oversight.
Challenges and Opportunities in the New Era
The transition to reasoning AI won't be easy. Several significant challenges lie ahead:
-
Developing robust reasoning algorithms: Designing algorithms capable of complex reasoning is a significant hurdle. Current methods often struggle with common-sense reasoning and handling ambiguity. We need new approaches that can effectively model human-like reasoning processes.
-
Data scarcity: While pre-training relies on vast datasets, reasoning AI may require more carefully curated, smaller datasets focused on specific reasoning tasks. Acquiring and annotating such data can be costly and time-consuming.
-
Explainability and interpretability: Understanding how reasoning AI systems arrive at their conclusions is crucial for trust and accountability. Developing methods for explaining the reasoning process is an ongoing challenge.
-
Generalization: Reasoning AI systems need to generalize well to new, unseen situations. This requires designing algorithms that are robust and adaptable to different contexts.
Despite these challenges, the opportunities are immense. Reasoning AI has the potential to revolutionize many fields, including:
-
Scientific discovery: AI systems capable of complex reasoning could accelerate scientific breakthroughs by analyzing complex data and formulating new hypotheses.
-
Healthcare: Reasoning AI could assist in diagnosing diseases, personalizing treatment plans, and accelerating drug discovery.
-
Finance: AI systems could improve risk management, fraud detection, and investment strategies.
-
Education: AI could personalize learning experiences and provide adaptive tutoring.
The Future is Now: Embracing the Reasoning Revolution
The end of the pre-training era isn't the end of AI; it's a new beginning. It's a call to embrace a more sophisticated, human-centered approach to AI development, one that prioritizes reasoning, understanding, and ethical considerations. It’s a shift from simply building machines that mimic human behavior to creating machines that truly understand.
This transition requires a collaborative effort from researchers, developers, policymakers, and the public. We need to invest in fundamental research, develop new algorithms, and establish ethical guidelines to ensure the responsible development and deployment of reasoning AI. The road ahead is challenging, but the potential rewards – a future where AI augments human intelligence and helps us solve some of the world's most pressing challenges – are immense.
This is not a transition we can afford to ignore. The future of AI hinges on our ability to move beyond brute force and embrace the power of reasoning. The future is now.
Frequently Asked Questions (FAQs)
Q1: What exactly does "pre-training" mean in the context of AI?
A1: Pre-training refers to the process of training AI models on massive datasets before fine-tuning them for specific tasks. Think of it as giving the model a broad education before specializing it. This approach has been dominant in recent years but is now being challenged.
Q2: Why is Ilya Sutskever's statement so significant?
A2: Sutskever is a highly respected figure in the AI community. His statement signifies a potential paradigm shift away from the current approach of relying solely on massive datasets and computing power towards a focus on reasoning and understanding.
Q3: What are the main limitations of the "pre-training" approach?
A3: Pre-trained models often lack true understanding and struggle with complex reasoning tasks. They excel at pattern recognition but fail to grasp the nuances of human thought.
Q4: What are some examples of reasoning AI applications?
A4: Reasoning AI could revolutionize healthcare (diagnosis, treatment), scientific discovery (hypothesis generation), finance (risk management), and education (personalized learning).
Q5: What are the biggest challenges in developing reasoning AI?
A5: Building algorithms for robust reasoning, securing sufficient data for training, ensuring explainability and interpretability, and achieving good generalization are all major obstacles.
Q6: How can we ensure the ethical development of reasoning AI?
A6: Ethical development requires a collaborative effort among researchers, developers, policymakers, and the public to establish guidelines, prioritize transparency, and address potential biases.
Conclusion
The assertion that the era of brute-force AI is ending marks a pivotal moment in the field's history. Sutskever's statement is a clarion call for a new era focused on reasoning, understanding, and ethical considerations. The transition will be challenging, demanding significant advancements in algorithmic design, data acquisition, and ethical frameworks. However, the potential rewards - a future of intelligent machines capable of true understanding and collaboration with humans – make this a challenge worth embracing. The future of AI is not just bigger models; it’s smarter models, and that future is now.
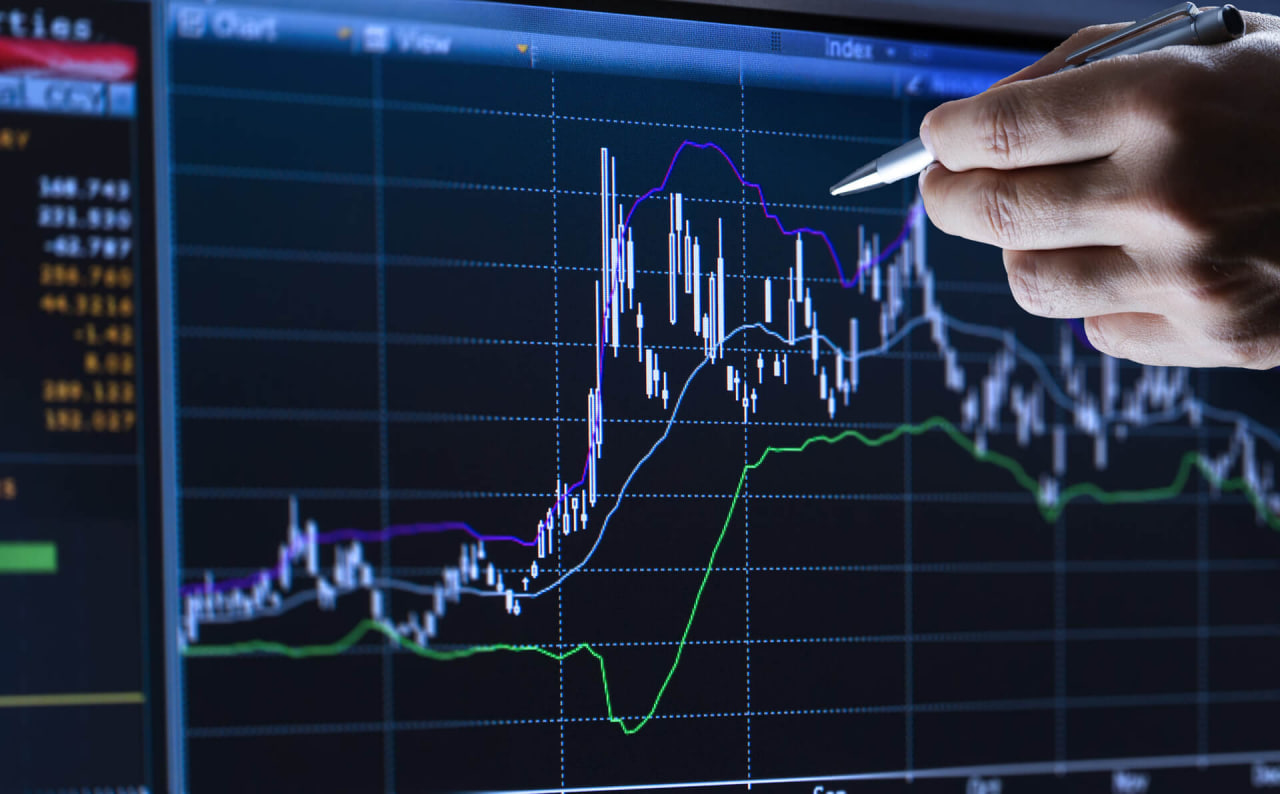